When we ask what are the characteristics of humanity that make humans unique, we have a tendency to look for qualities in humans that we can observe with our senses. For example, morphological or physiological characteristics, such as bipedalism or our big brain-to-body weight ratio are good candidates to consider as uniquely human. Language is another good candidate. Some say it is our ability to lie. Others argue that what distinguishes humans from other life forms is the development and use of tools, or technology. But all of these can be found in some form or another, to a higher or lesser degree, in other life forms.
The intersection of two unexpected fields, physics and urban studies, has resulted in new ways to look at this question suggesting that what defines humanity cannot be seen directly. Rather, it is hidden in the abstract mathematical probability distributions that we use to describe cities.
While urbanization is a relatively recent phenomenon in human history, cities offer a magnifying lens to understand interactions between human beings and their behaviour. Tools from physics allow us to analyse, characterize and understand what we see with these lenses.
But physics is not alone in this journey. With larger and more detailed data sets about cities becoming increasingly available, other disciplines like computer science and complex networks science are increasingly applying their own tools. These new approaches share some differences with the traditional ones.
While traditional fields such as regional sciences, urban economics, geography, urban planning, architecture and engineering have been mostly prescriptive, such new approaches are more about scientific understanding, focusing on empirical regularities in cities that are leading to new methods, concepts and theories about human behaviour. The success of these new approaches ultimately hinges on whether we will be able to bring all the findings together to form a unified theory of social systems.
Searching for Universals
Regarding the question of what makes humans unique, physics has a lot to say thanks to its idiosyncratic style of inquiry. The biophysicist William Bialek from Princeton University, in his book Biophysics: Searching for Principles, argues that “[a]cademic disciplines have a choice to define themselves either by their objects of study or by their style of inquiry. Physics… is firmly in the second camp.”1 Physics’ style of inquiry is to look for universal principles that apply to a wide range of phenomena, which can then be expressed in the precise language of mathematics. According to this dictum, those who seek universals are following the physics style of inquiry, regardless of their diploma.
The study of human systems through a physics lens is thus a search for universals. You may think, however, that there are no universals when studying humans: there are too many different behaviours, different cultures, and contingencies. You may grant that there are certainly some generalities, yes, but never universals. Allow me to challenge that position. The sub-discipline that gives us the best tools to study cities is called statistical physics.
Statistics is a complex and sometimes murky discipline. In fact, there are two (not incompatible) takes on statistics. On the one hand, you can use it as a way to substitute a stream of raw data with a short summary of it. In this way, statistics is a tool as much as it is a need, given our mental limitations on understanding but a few pieces of information at a time. This is why statistics is so powerful and important, but also sometimes pretty boring. According to this take, you use statistics not because you like data, but actually because you don’t. Hence, you want a short description of the data - a simplification of it.
But statistics can be used differently. Statistics, in another sense, can be used to understand the internal mechanics of the system you have been measuring (in our case, cities). One key statistical idea that can be used for this is the concept of a probability distribution function, or PDF.
PDFs as a mathematical object were first developed in the beginnings of eighteen century by Jacob Bernoulli and Abraham de Moivre (for a highly entertaining history of probability theory I recommend reading a book by Peter Bernstein2). But it wasn't until the middle of nineteen century that PDFs were used to make a link between microscopic dynamics and macroscopic behavior. PDFs provided our first understanding of what gases are, and why they have the properties that they have. Remember “Phony Vampires are not Real Things”, or PV=nRT? That empirical fact can be traced back to a PDF. It happened in 1867 when James Clerk Maxwell (the Scottish physicist that established in 1873 our first complete understanding of the phenomena of electricity, magnetism and light, with just four equations) published a paper3 showing how the properties of gases had to be the result of small particles interacting with each other, changing each other’s velocities, and moving erratically from place to place (at that point in history the existence of atoms and molecules was being fiercely debated). However, tracing the paths of all these particles is next to impossible. The link between what happens at the level of particles with what we end up observing, for example, at the level of a balloon of gas, is not trivial. Maxwell's trick was to characterize all the jiggling of the particles through a single mathematical function, and this function is the PDF of the velocities of the particles.
You probably can see what I am getting at.
What I’m getting at is that we do not want to understand what is truly unique about humans by simplifying and summarizing our behaviour through a few statistics. We want to use statistics, in the second sense: to understand how we behave, and how we interact with each other. Thus, we need to identify all the probability distributions that describe the behaviour of individuals, as well as the behaviour of teams of individuals, up to the scale of cities.
Since Maxwell, we have been increasing our understanding of PDFs to inform us about which processes may have generated the stream of data that is observed.4
So what have we learned so far about cities from their PDFs?
Cities, interactions and complementarity
We are so embedded in our daily routine that we sometimes lose focus of how strange our life really is, as compared to not only other animals, but to our ancestors. The fact that it is very likely that you are reading this in a city is actually pretty astonishing, and pretty unique.
Cities are unexpected from an evolutionary point of view. Evolution seems to have come up with a rule of thumb that says “don’t interact with strangers”. In fact, it is rather something like “kill it, or better yet, eat it”. Yet, cities are big agglomerations of genetically unrelated individuals, interacting in positive ways most of the time (not eating each other, and although killings sometimes occur, they are becoming less and less frequent5). This is a phenomenon that stands as uniquely human. But how do we know that interactions between individuals are a key feature of cities? How do we know the effect of human interactions do not wither beyond and above a certain range?
There are many ways, of course, but one way is through a PDF that describes measures of total output of cities. Examples of measures of total output include the total number of patents that originate in a city (over a period of time), or the size of its economic production (i.e., the Gross Domestic Product, or GDP), but also the total number of homicides. From an accounting point of view, the total output of a city is the total sum of what all the many individuals (or firms) produce in the city.
At this point, a key piece of knowledge from the statistics of PDFs enters the story. From what mathematicians call the “Central Limit Theorem”, we know very well the shape of the PDF of a sum of many values when the elements of the sum are independent of one another. It is a mathematical truth that applies to all sums of independent random variables, regardless of the context. Guess what - the PDF of urban total output does not have that shape (the shape I am referring to is the famous Gaussian distribution). Ergo, the elements of the sum that compose urban output are not independent. Or put more explicitly, the components of cities are strongly interdependent entities.
Leon Tolstoy’s book Anna Karenina begins with the following assertion: “Happy families are all alike; every unhappy family is unhappy in its own way”. This sentence can be taken as a principle. It applies in those situations in which no single and independent factor guarantees the successful occurrence of an event (e.g., being a happy family), yet the absence of any guarantees failure (e.g., being an unhappy family).
Beyond being not Gaussian, the shape of the PDF of total urban output that we mentioned above suggests, more specifically, that production processes in cities arise from a large multiplicity of factors being complementary to each other. In other words, production processes in cities tend to follow Anna Karenina’s Principle, or AKP.
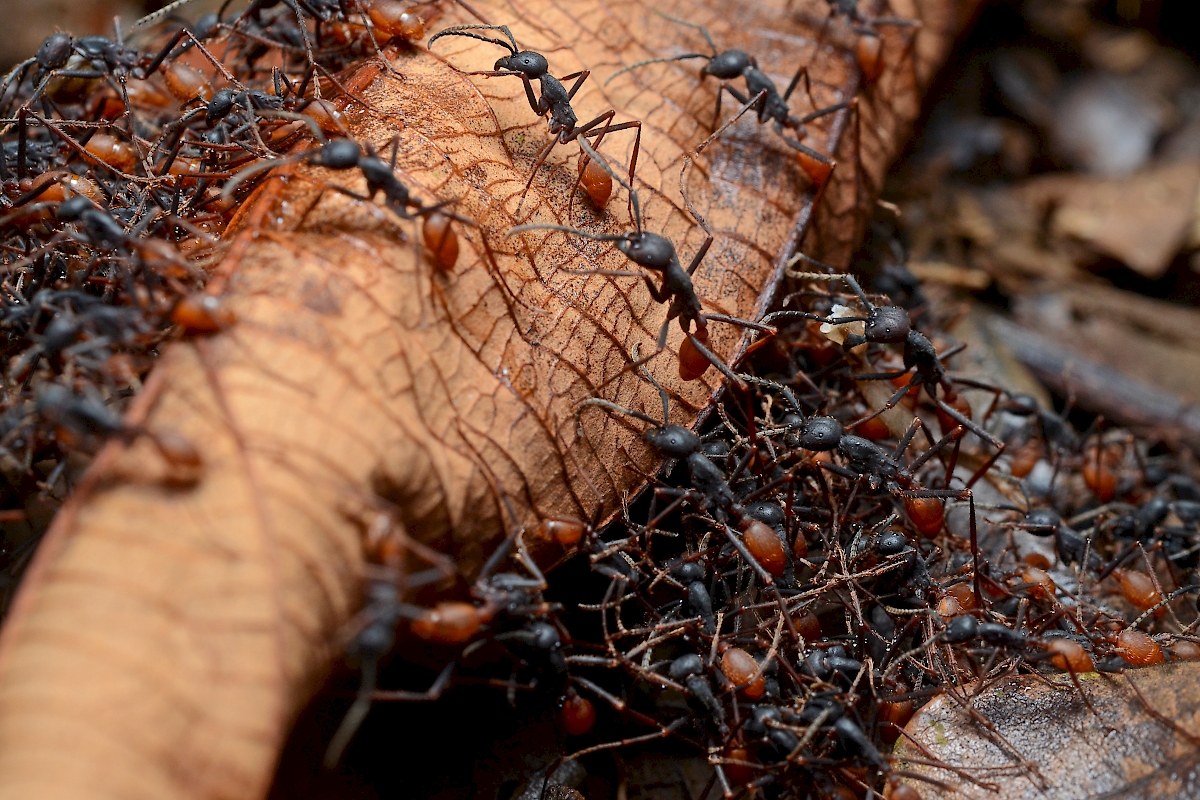
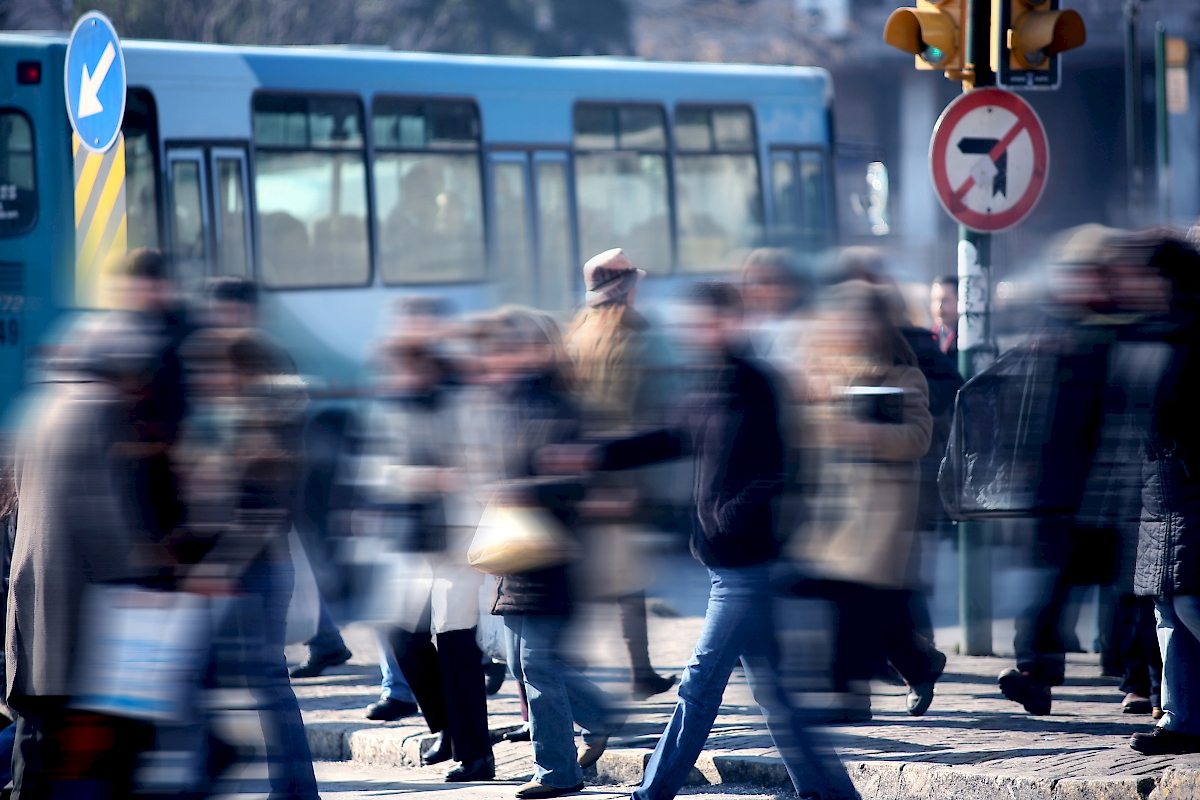
Thus, AKP constrains the development of cities as a whole. It explains why, in general, poor cities remain poor and rich cities get richer. If we are talking about innovation, for example, it explains why Silicon Valley is so unique and why it has been so hard to replicate elsewhere. Notice, however, that the AKP as applied to this context is a property of the process of innovation in itself. Not a direct property of individuals.
It seems to be the case that most urban activities, like landing a job, acquiring a patent, getting sick, or obtaining a university degree, follow this principle. However, some activities require a larger number of factors than others. In other words, some activities are more difficult than others. But as cities get bigger, their populations are spontaneously able to support the generation of more difficult activities. This implies two things: First, larger cities generate larger diversity of increasingly complex activities. Second, teams of humans are able to coordinate their efforts in increasingly larger groups, in a seemingly unbounded way, when the conditions are right. This should strike you because it is a statement of the incredibly flexible manner in which humans interact.
The flexibility and long-range effect of interactions may be connected to one of the most elusive features about cities: their wide differences in size. People have known for a long time (at least since the beginnings of twentieth century) that the PDF that describes city sizes establishes that city sizes have no scale. Regarding the size of cities, in other words, there is no such thing as an “average” city. Remember that PDFs informs us about the process that generated the data. In this case, it says that the proportion in which a city grows over a given period of time is the same regardless of whether the city is small or large. But cities are also very dense, and this means that the spatial length of the interactions, as compared to the overall scale of cities, is very short, very localized.
The well-intended mistake of economics
What we have been saying so far should give you a sense of why humans are so diverse, and why social systems are so heterogeneous. Not much constrains humans, since they are able to interact widely with others, and satisfy their needs from these diverse interactions. And since these interactions are so flexible and strong, humans can team up in very large localized groups which accomplish very complex activities.
This view, however, is in blunt disagreement with the view portrayed by economic models of human societies. These models seek to portray representative individuals, representative firms, and representative cities. Moreover, with their empirics, economic models constantly seek to identify representative causes of representative phenomena. Economics as a discipline has overemphasized statistics in the first sense, over-summarizing and oversimplifying. In short, this view misses exactly what humans are about.
This misrepresentation of humans has been, of course, well-intended. There has been confusion between doing science and searching for common denominators. These two have overlapped for a long time in the early history of physics. Indeed, physics advanced greatly by seeking the common physical denominators to all things (namely, that everything is made of a few fermions which interact through a few bosons). But this approach is less appropriate in biology, and arguably even less so in social systems. This means that economics has to tackle the complexity of its systems of study directly. Diversity and heterogeneity are inherent properties of humans that should not be summarized by representative agents and average properties.
What is unique to humans, hence, becomes evident not at the level of individuals, but at the level of societies. What we learn at this societal level is that humans are highly versatile in their interactions with others. Our emerging understanding of cities links back to studies in anthropology that say we are a super-cooperative species.6 That is, physiological characteristics do not distinguish us sharply from other animals. But our social organizations do. And what makes these huge agglomerations of humans beautiful is their stunning diversity and complexity.